Artificial Intelligence (AI) and Machine Learning (ML) are two of the most transformative technologies of our time. While often used interchangeably, these terms represent distinct concepts with unique applications. Understanding the differences between AI and ML is crucial for leveraging their potential in business, research, and daily life. We hope this article will help you clearly understand the AI vs Machine Learning concept so you can be confident when using it in your workplace.
What is Artificial Intelligence?
AI refers to the simulation of human intelligence in machines programmed to think, learn, and make decisions. It encompasses a broad range of technologies, from simple rule-based systems to complex neural networks.
Key Characteristics of AI:
- Automation: AI systems can automate tasks traditionally requiring human intervention.
- Reasoning: AI can make decisions based on data and predefined rules.
- Adaptability: Advanced AI systems learn and adapt over time.
Examples of AI in Action:
- Chatbots providing customer service.
- Autonomous vehicles navigating roads.
- Recommendation systems on platforms like Netflix and Amazon.
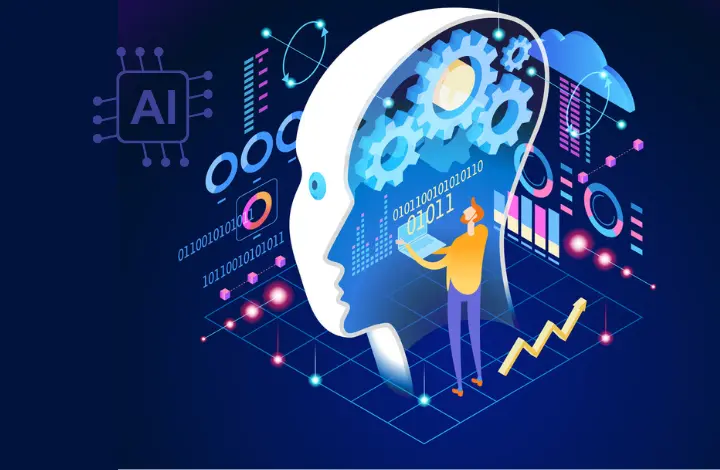
What is Machine Learning?
ML is a subset of AI that focuses on enabling machines to learn from data without explicit programming. Using algorithms, ML models improve their performance as they process more data.
Key Characteristics of ML:
- Data Dependency: ML requires large datasets for training.
- Algorithm-Driven: It relies on statistical methods and algorithms to identify patterns.
- Iterative Improvement: ML models continuously refine their accuracy through feedback.
Examples of ML in Action:
- Email spam filters distinguishing between legitimate and spam messages.
- Predictive analytics in finance and healthcare.
- Image recognition in security and social media platforms.
AI vs Machine Learning: Key Differences
Aspect | Artificial Intelligence | Machine Learning |
---|---|---|
Definition | The broader concept of machines mimicking human intelligence. | A subset of AI focused on learning from data to make predictions. |
Scope | Encompasses various techniques, including ML, expert systems, and robotics. | Limited to data-driven learning and prediction. |
Autonomy | Can function with or without ML. | Requires AI frameworks to operate effectively. |
Applications | Autonomous systems, conversational AI, decision-making tools. | Pattern recognition, forecasting, recommendation systems. |
Why Understanding the Difference Matters
In today’s technology-driven world, the distinction between AI and ML is more than just semantics. Knowing how these technologies work can guide decisions in:
- Business Strategy: AI and ML can optimize operations, enhance customer experiences, and drive innovation. For example, AI-powered chatbots can automate customer support, while ML algorithms can analyze user data to predict trends.
- Career Opportunities: Professionals skilled in AI and ML are in high demand. Understanding their nuances can open doors to careers in data science, software engineering, and AI research.
- Ethical Considerations: AI and ML technologies raise ethical questions around data privacy, decision-making transparency, and bias. Awareness of their capabilities and limitations is essential for responsible use.
Real-World Examples: AI and ML Working Together
Many applications combine AI and ML to deliver seamless functionality. For instance:
- Voice Assistants: AI enables natural language understanding, while ML improves voice recognition accuracy.
- E-commerce: AI powers personalized shopping experiences, while ML predicts customer preferences.
- Healthcare: AI aids in diagnostics, while ML analyzes patient data to suggest treatments.
Conclusion
While AI and Machine Learning are interconnected, their distinctions are vital for understanding the evolving tech landscape. AI represents the broader goal of creating intelligent machines, while ML is a critical tool that enables AI systems to learn and adapt. By grasping their differences and synergies, businesses and individuals can harness their full potential to drive innovation and solve complex challenges.
Leave a Reply